Transparency and explainability in image classification are essential for establishing trust in machine learning models and detecting biases and errors. State-of-the-art explainability methods generate saliency maps to show where a specific class is identified, without providing a detailed explanation of the model’s decision process. Striving to address such a need, we introduce a post-hoc method that explains the entire feature extraction process of a Convolutional Neural Network. These explanations include a layer-wise representation of the features the model extracts from the input. Such features are represented as saliency maps generated by clustering and merging similar feature maps, to which we associate a weight derived by generalizing Grad-CAM for the proposed methodology. To further enhance these explanations, we include a set of textual labels collected through a gamified crowdsourcing activity and processed using NLP techniques and Sentence-BERT. Finally, we show an approach to generate global explanations by aggregating labels across multiple images.
The pipeline showing the process is shown below. In the first step, feature maps and their weights are extracted from the CNN. These feature maps are clustered to generate cluster maps. Subsequently, labels are collected through crowdsourcing and processed using NLP techniques. Finally, cluster maps with the same top label are merged.
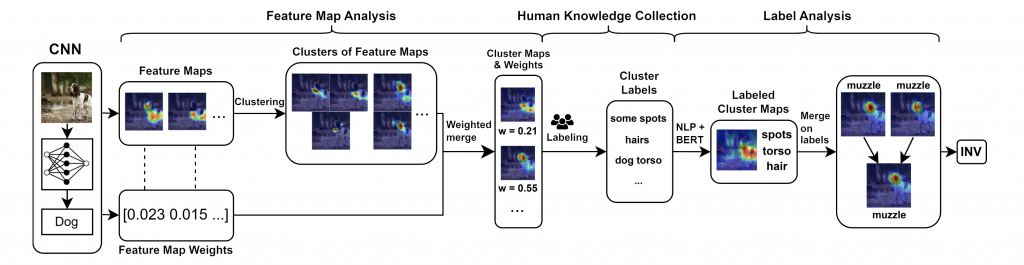
As an example of generated explanation, here you can see the result for an image of the class “church”. The most important feature for this prediction was “steeple”. However, it can be observed that other elements, such as “cross” and “roof” also contributed to the classification.
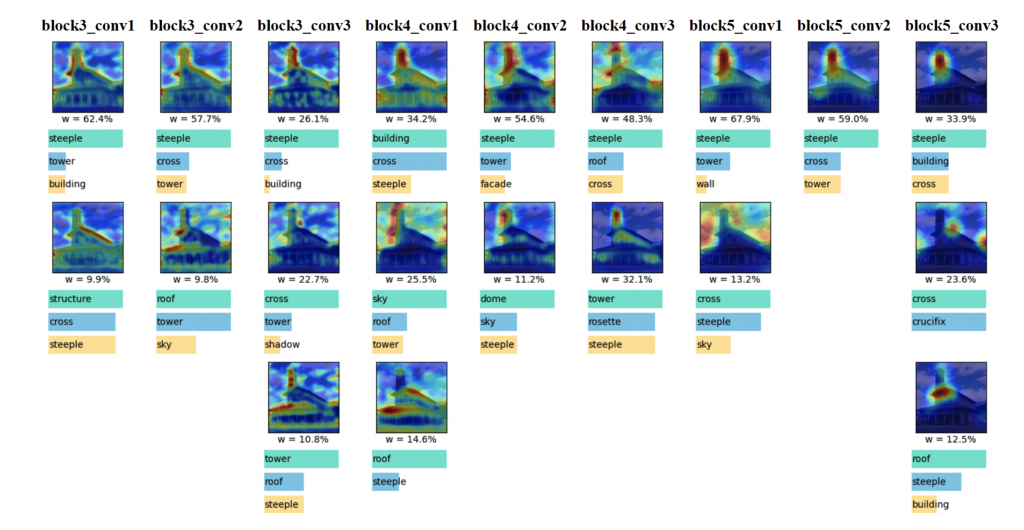
This work is published at IJCAI as: